Predictive analytics
Predictive analytics, a way to anticipate the future with new technologies
Predictive analytics uses statistical modelling techniques, big data and machine learning to extract historical data and make predictions. In the business world, it is a highly valued technique because of the benefits it can bring in terms of identifying risks and opportunities, for example. Its application ranges from e-commerce to energy, finance and insurance, among others.
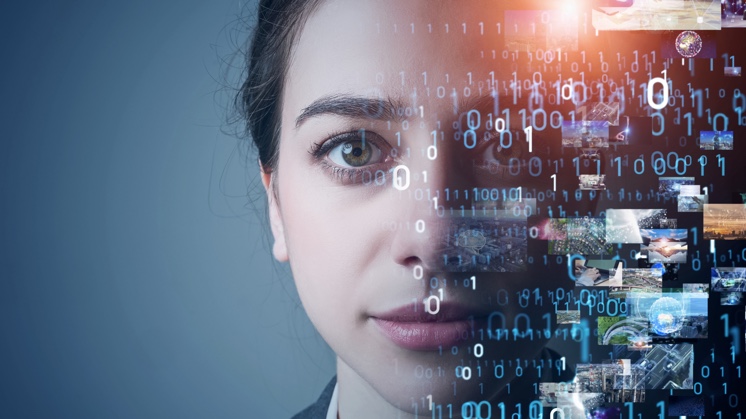
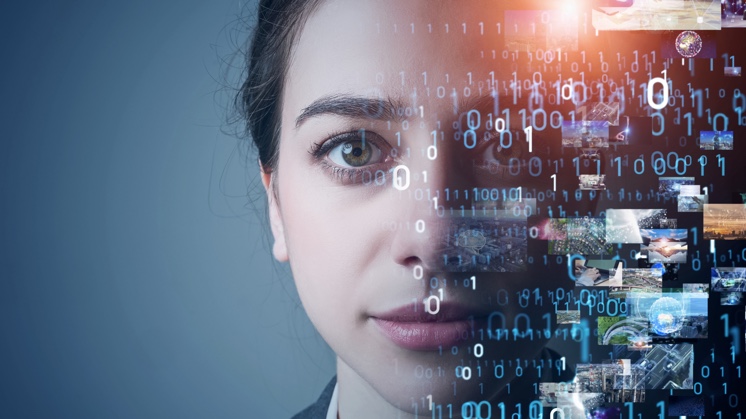
Predictive analytics is very useful for companies, as it allows them to anticipate the demands of their customers.
One day you are thinking about buying some new ski boots for your next trip to the mountains and you receive an email with an offer. When something like this happens, you have probably asked yourself on more than one occasion: are they reading my mind? In a way you could say yes, because events like this do not happen by chance, they are a result of predictive analytics.
What is predictive analytics
According to consultancy firm Gartner, predictive analytics is a form of advanced analytics that examines data or content to answer the question: what is likely to happen in the future? Thanks to big data, information from all connected systems can be interpreted to make predictions about how a person or a group of people will behave, which can also be applied to businesses or processes.
There are differences between descriptive, diagnostic, predictive and prescriptive analysis, but we will now focus on the latter two. While predictive analytics uses data collected to make predictions about an assumption, prescriptive analytics takes that data and delves deeper to find a way to make that assumption happen. With respect to the first two, descriptive analysis examines the event itself, while diagnostic analysis deals with the causes.
What are the different types of analysis and what are they for?
The greater the difficulty of an analysis, the greater the value of the information extracted.
Value
Difficulty
- How can we make it happen? Prescriptive analysis
- What will happen? Predictive analysis
- Why did it happen? Diagnostic analysis
- What has happened? Descriptive analysis
- Hindsight Retrospective metrics are gathered to see what has happened.
- Insight Causes are analysed to understand patterns of behaviour.
- Foresight The aim is to predict what is most likely to happen in the future.
Source: Gartner.
SEE INFOGRAPHIC: What are the different types of analysis and what are they for? [PDF] External link, opens in new window.
Predictive models
Predictive modelling is a statistical model that evaluates data, detects patterns and uses that information to predict future trends. There are two classes, parametric and non-parametric, and a third, semi-parametric, which lies between the two. Parametric models make assumptions about a finite number of parameters analysed within a family of distributions. In contrast, non-parametric models study tests whose distribution does not fit parametric criteria.
How to do predictive analytics?
The process of predictive analytics starts from a dataset and requires the following steps:
1. Project definition
Identify the objective of the project and the deliverables to achieve a result.
2. Data collection
Collect data from multiple sources to obtain the most complete picture possible.
3. Data analysis
Data are inspected, sieved and modelled to draw conclusions.
4. Statistic development
The aim is to validate whether the conclusions, assumptions and hypotheses are consistent.
5. Creation of the predictive model
A predictive model that allows parameters to be modified to modulate the results.
6. Deploying the predictive model
Use the results to generate reports and metrics, and make decisions based on them.
7. Follow-up the results
Subsequently, check the results to see if they are in line with the predictions obtained.
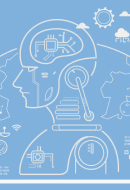
What is Artificial Intelligence?
Are we aware of the challenges and main applications of Artificial Intelligence?
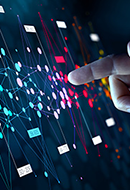
AI Algorithms
Discover the types of algorithms that AI uses.
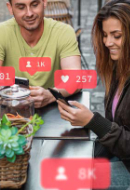
Internet of Behaviour
The capacity for data collection makes it possible to obtain individual patterns on a massive scale.
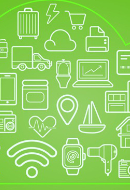
Internet of Things (IoT)
Are we ready for the world that new technologies will bring?
Benefits of predictive analytics
The main benefit of predictive analytics is that it allows companies and organisations to learn from their past experience from their data and take action to apply what they have learned in the future to achieve better results. In addition, we review some of its other advantages:
Predictive analytics techniques
The predictive analytical techniques used for modelling are based on mathematical methods for forecasting future events and outcomes. Some of the most common are: linear regression, discrete choice, time series, survival or duration analysis, classification and regression trees, etc. There are also a number of advanced statistical methods for regression and classification using machine learning techniques. These are some of the best known:
- Gradient boosting: a machine learning technique used for regression analysis and statistical classification problems that produces a model in the form of decision trees.
- Bayesian analysis: it is based on subjective probability and works by considering knowledge prior to an investigation plus the evidence obtained from it.
- Artificial neural networks: mimics the functioning of the human brain and its ability to learn or adapt, to organise or generalise data.
- Random forest: allows the construction of a set of decision trees with the idea that a combination of learning models will improve the overall outcome.
- Data mining: this computational technique consists of exploring, analysing and linking large blocks of information to obtain meaningful patterns and trends.
Predictive analytics tools
Business intelligence software solutions incorporate predictive analytics tools. These services employ sophisticated techniques to collect company data and perform statistical analysis and add machine learning to make projections about the future.
Some of the most common tools on the market are SAP Analytics Cloud (part of the SAP environment), RapidMiner (has free options), Alteryx (for teams), IBM SPSS (for research), H2O.ai (open source), TIBCO (offers a free trial version), MathWorks (part of the MathLab environment), DASH Analytics (for healthcare).
Applications of predictive analytics
Predictive analytics is used successfully in many sectors. In the following lines, we review some of them and cite some examples: